

This table shows the adjusted mean for each product-descriptor combination. Graphics for each product with the coefficients are then displayed. Adjusted mean, t test, p-value and confidence interval for each combination are also displayed. This table displays the various coefficients of the chosen model for each combination product-descriptor. Associated V-test and p-values are also displayed. This table shows the ordered descriptors from the most discriminating on the products to the least discriminating. Results of XLSTAT for product characterization Discriminating power by descriptor Product characterization is a very efficient tool to characterize products using judges’ preferences. It means we consider each judge to have its own way of giving scores to the products (on the score scale). The judge effect is always supposed to be random. Score = product effect + judge effect + product effect * judge effect We then can test if some combines of the judges and products are giving higher or lower grades on the descriptors. Score = product effect + judge effect + session effectĪn interaction factor can also be included. If different sessions have been organized (each judge has evaluated at least twice each product), the session factor can be added and the model becomes: The dataset must contain the following columns: one identifying the judge, one identifying the product, eventually one identifying the session, and as many columns as there are descriptors or characteristics.įor each descriptor an ANOVA model is applied to check if the scores given by the judges are significantly different. Each row should concern a given product, eventually a given session and should gather scores given by a judge for one or more descriptors associated to the designated product. Computation for product characterizationĪll computations are based on the analysis of variance (ANOVA) model. You can also identify which are the most important characteristics of each product. Rapid Communications in Mass Spectrometry Wiley Product characterization provides the XLSTAT users with a user-friendly analytical method that helps finding in a sensory study which descriptors are discriminating well a set of products. Limited water availability is one of the main factors restricting crop production (Boyer, 1982 Tollenaar and Lee, 2002) and it is predicted to become an increasing problem under future climate conditions (Burke et al., 2009 Lobell et al., 2011). Conclusions Discrimination techniques for side‐window glass, although not for side mirrors, using chemical compositions combined with multivariate statistical analyses provide evidence for forensic investigations. However, glass from side mirrors cannot be used for good forensic identifiers. From the LDA, the types of side‐window glass were successfully discriminated according to car manufacturer, glassmaker, and even glass thickness. In contrast, light rare earth elements (LREEs) were different from each glassmaker.
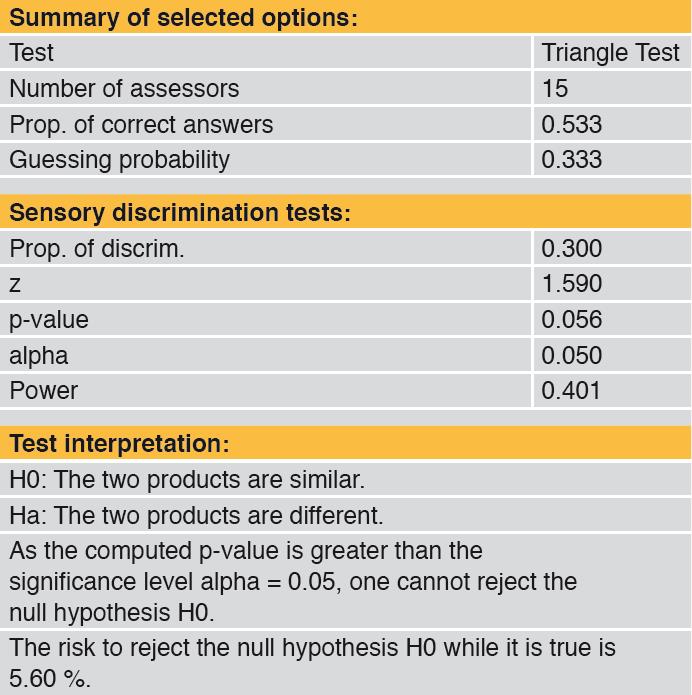
Results The percentages of major elements (Si, Ca, and Fe) in side‐window glass varied within narrow ranges (30.0 ± 2.36%, 5.93 ± 0.52%, and 0.33 ± 0.05%, respectively), while the differences among Pb isotope ratios were not significant. Their chemical compositions (including Pb isotopes) were analyzed using laser ablation inductively coupled plasma mass spectrometry (LA‐ICP‐MS) and linear discriminant analysis (LDA) was performed. In addition, 120 samples from side mirrors were collected from the same suppliers as well as from small businesses.
Discriminate in xlstat windows#
Methods Thirty‐five samples from the side windows of cars produced and used in South Korea were collected from the official agencies of five car manufacturers and from two glassmakers. Our results represent a promising approach to a thorough forensic investigation of car glass. By identifying the origin of glass fragments, it is possible to infer the identity of a suspect. The glass fragments are important as trace evidence at crime scenes related to car accidents and burgled homes. Rationale Fragments of glass from cars are often found at crime scenes and can be crucial evidence for solving the crime. Lee, Sin‐Woo Ryu, Jong‐Sik Min, Ji‐Sook Choi, Man‐Yong Lee, Kwang‐Sik Shin, Woo‐Jin Discrimination of side‐window glass of Korean autos by laser ablation inductively coupled plasma mass spectrometry Discrimination of side‐window glass of Korean autos by laser ablation inductively coupled plasma.
